Cherry tomato (Solanum lycopersicum) is popular with consumers over the world due to its special flavor. Soluble solids content (SSC) and firmness are two key metrics for evaluating the product qualities. The existing measuring techniques relying upon chemistry reactions can derive the SSC value accurately. However, the destructive methods can not be applied in high volume measurements. Moreover, there are significant variations so that sampling can be inefficient and inaccurate. Hyperspectral imaging technology can simultaneously detect the two-dimensional spatial information and 1D spectral information, therefore combine image and spectral characteristics. It has been widely used for nondestructive testing in various fields, such as detection of plant disease stress, industrial food packaging, medical image classification, horticultural products, and quality analysis of fruits.
Based on this, in the attached article, a group of scientists from Zhejiang University of Technology and Zhejiang Academy of Agricultural Sciences chose a local mainstream cherry tomato (cultivar: Zheyingfen-1) as a research object, measured the baseline firmness and SSC of cherry tomatoes and developed non-destructive testing techniques for SSC and fruit firmness based on hyperspectral images (PIKA XC hyperspectral camera, Resonon Inc., Bozeman, MT, USA) and the corresponding deep learning regression model.
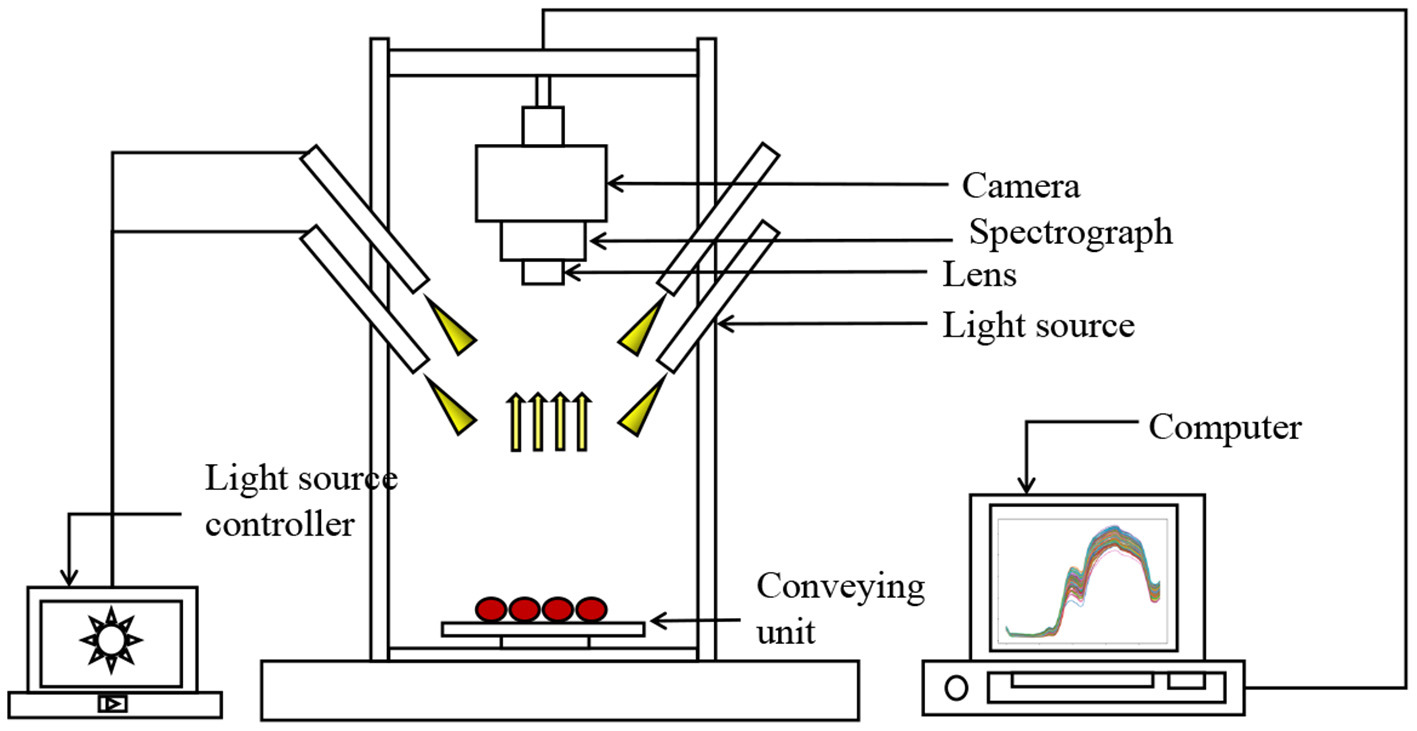
Schematic of the hyperspectral imaging system for acquiring spectral scattering images from cherry tomatoes.
Results:
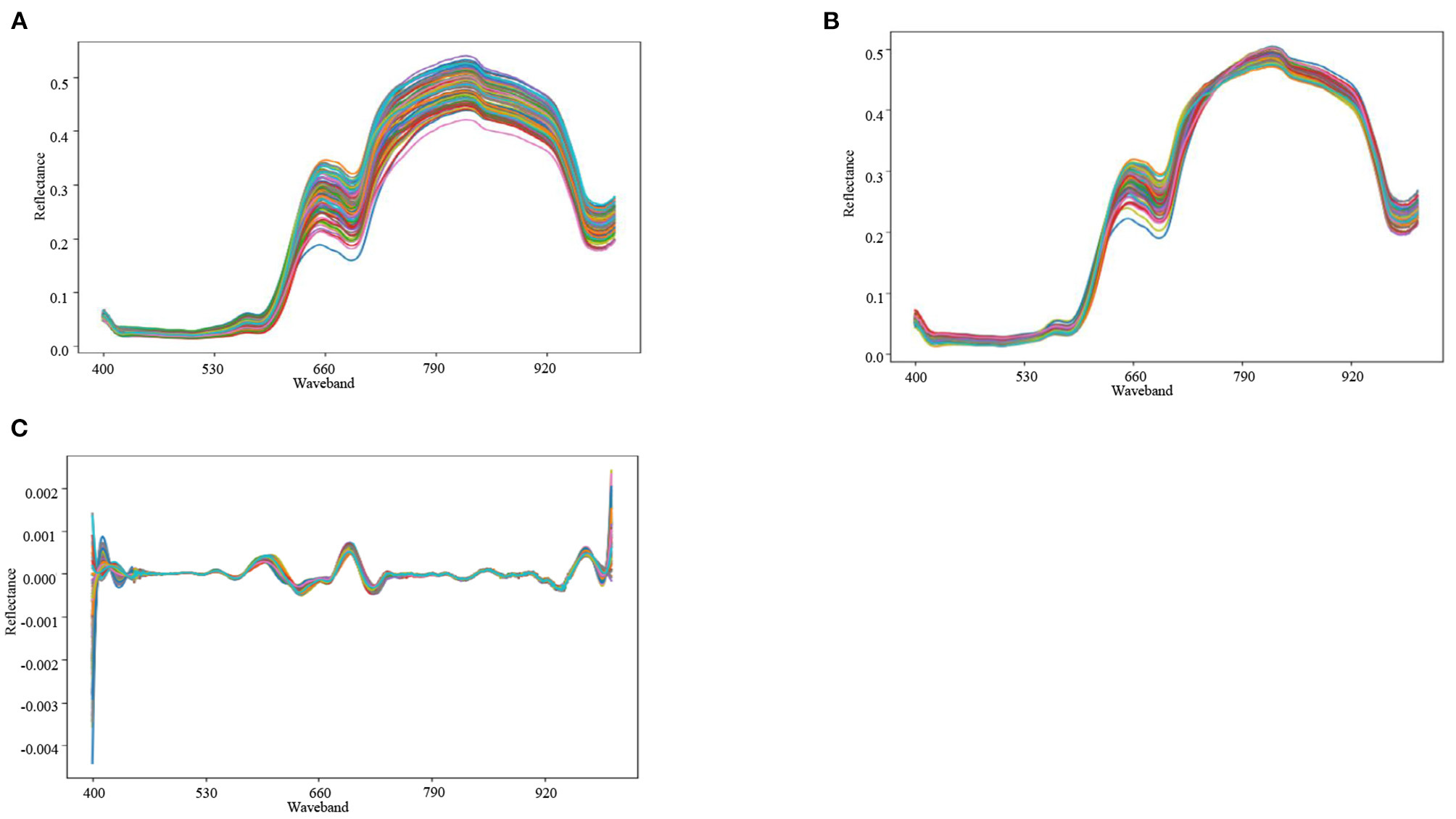
Corrected spectral reflectance map. (B) MSC preprocessing. (C) Second-order differential preprocessing.
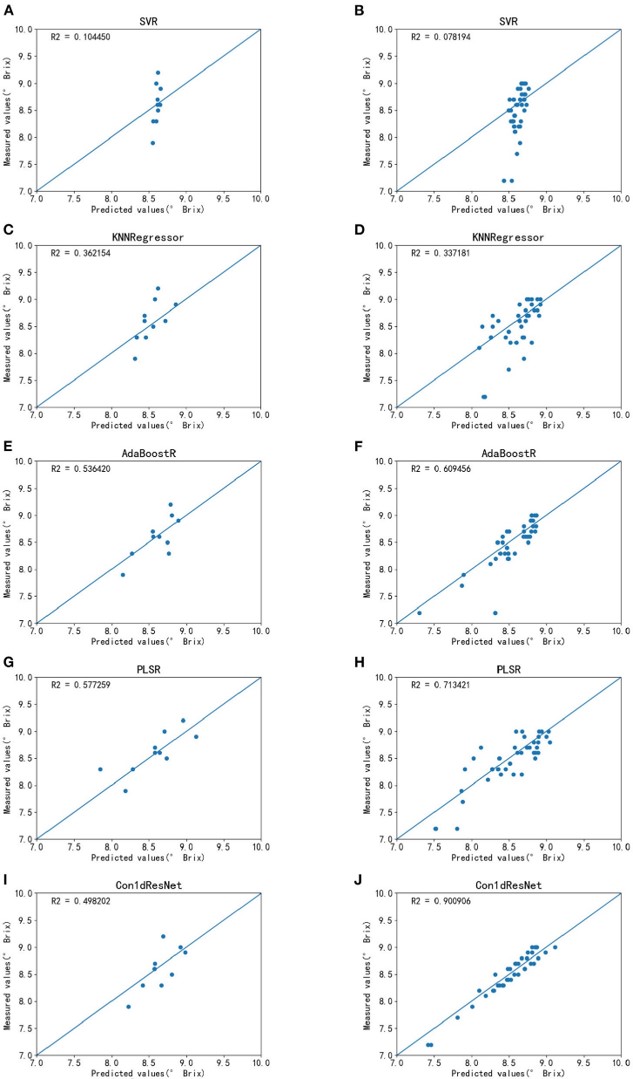
SSC estimation results for each model. (A) SVR estimation results on small sample data. (B) SVR estimation results on large sample data. (C) KNNR estimation results on small sample data. (D) KNNR estimation results on large sample data. (E) AdaBoostR estimation results on small sample data. (F) AdaBoostR estimation results on large sample data. (G) PLSR estimation results on small sample data. (H) PLSR estimation results on large sample data. (I) Con1dResNet estimation results on small sample data. (J) Con1dResNet estimation results on large sample data.
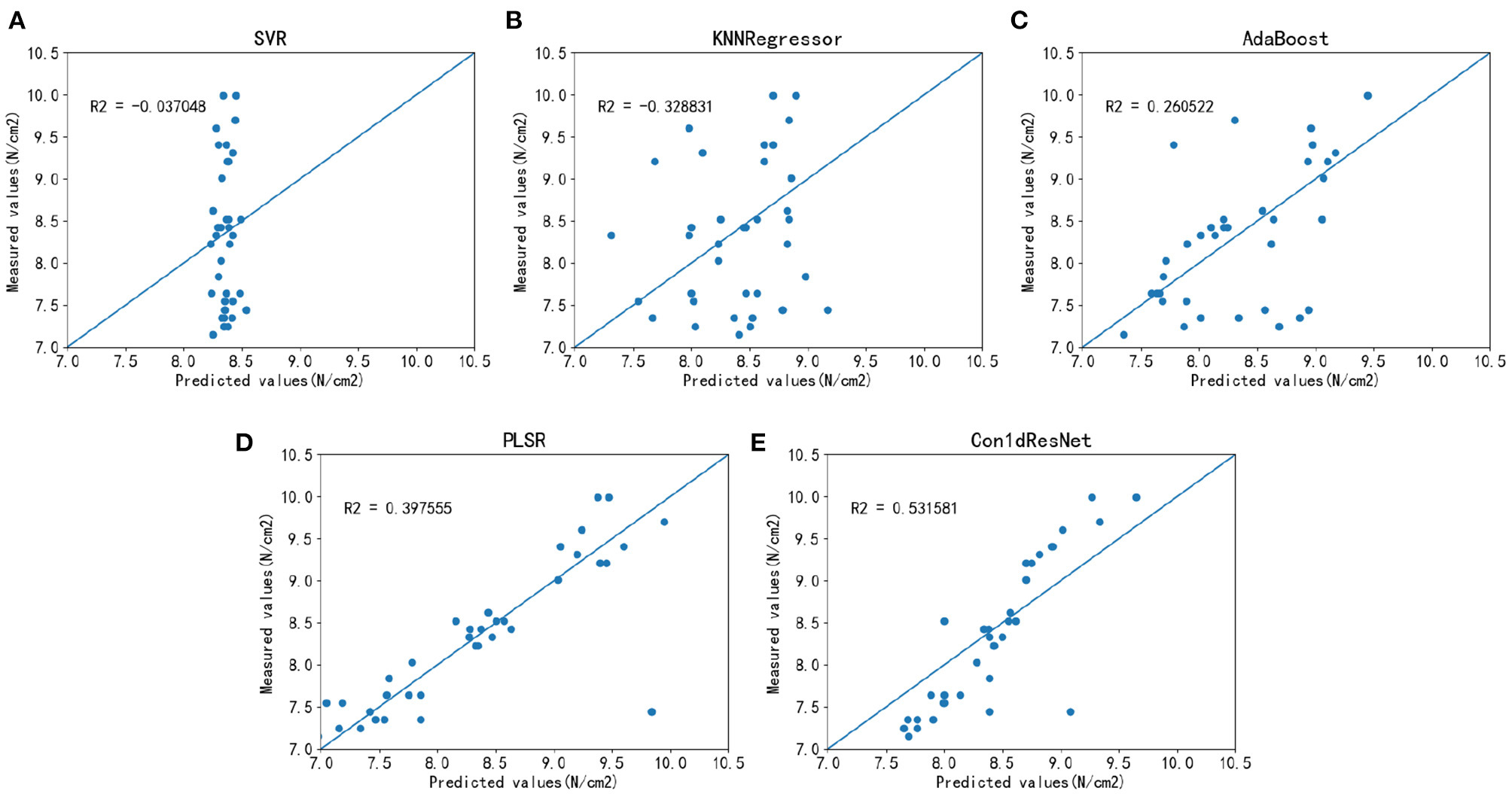
Estimation results of firmness for each model on a large sample dataset. (A) SVR. (B) KNNR. (C) AdaBoostR. (D) PLSR. (E) Con1dResNet.
Conclusions:
In this work, the authors propose Con1dResNet, a deep learning based technique, to estimate the SSC and firmness of cherry tomatoes using hyperspectral images. With sufficient sample size, it can achieve better results than traditional machine learning methods. For SSC estimation, its R2 value is 0.901, which is 26.4% higher than PLSR, while its MSE is 0.018, which is 0.046 lower than PLSR. For Firmness estimation, its R2 value is 0.532, which is still 33.7% better than PLSR. The results indicate that hyperspectral imaging combined with deep learning can significantly improve the cherry tomato SSC and firmness estimation accuracies.