The pine wood nematode (Bursaphelenchus xylophilus) is a dangerous invasive species that causes pine wilt disease (PWD), which has already destroyed enormous areas of pine forest in China. Therefore, early-stage PWD detection is crucial for a prompt management response. Hyperspectral RS uses data from hundreds of bands and continuous wavelengths, and these bands can capture physiological changes in infested trees, which help detect early-stage pest and diseases infestations. Unmanned aerial vehicle (UAV)-based hyperspectral images (HSI) can detect tree-level changes and are thus an effective tool for forest change detection. However, previous studies mainly used single-date UAV-based HIS data, which could not monitor the temporal changes of disease distribution and determine the optimal detection period.
Based on this, in the attached article, a group of scientists from Beijing Forestry University conducted study using multi-temporal data in Dongzhou District, Fushun City, Liaoning Province, northeast China (124°12′36′′ -124°13′48′′ E,41°56′53′′ -41°57′46′′). During an outbreak of PWD, field surveys were carried out six times: on 9 May, 9 June, 11 July, 11 August, 13 September, and 21 October 2021 (Through morphological and molecular identification, 59 trees were confirmed to be infected with the B. xylophilus, the same number of healthy trees were selected as control). A DJI Matrice 600 Pro UAV equipped with a Pika L hyperspectral camera (Resonon, Bozeman, MA, USA) and a LR1601-IRIS LiDAR system (Beijing LICA-IRIS Inc., Beijing, China) were used to acquire HSI and LiDAR data from 11:50-12:30, on 11 May, 10 June, 12 July, 18 August, 15 September, 23 October 2021 under sunny and cloudless weather conditions. UAV RGB images were collected synchronously under the same conditions. Spectral features of each tree crown, such as spectral reflectance, first and second-order spectral derivatives, and vegetation indices (VIs), were analyzed to identify those useful for early monitoring of PWD. The Random Forest (RF) classification algorithm was used to examine the separability between the two groups of trees (control and infested trees).
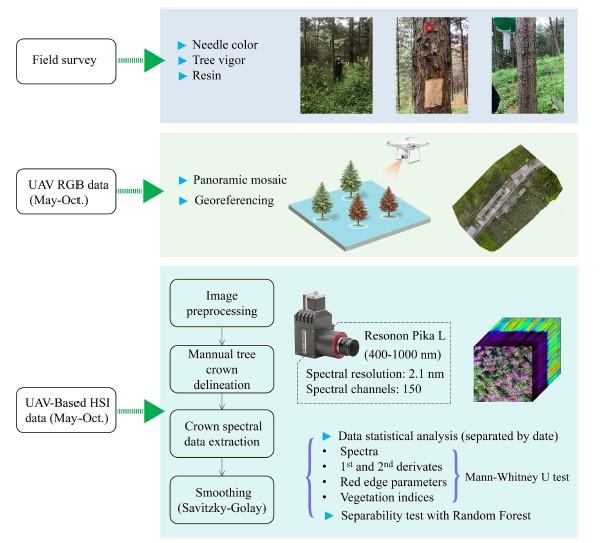
Flowchart of data acquisition and processing.
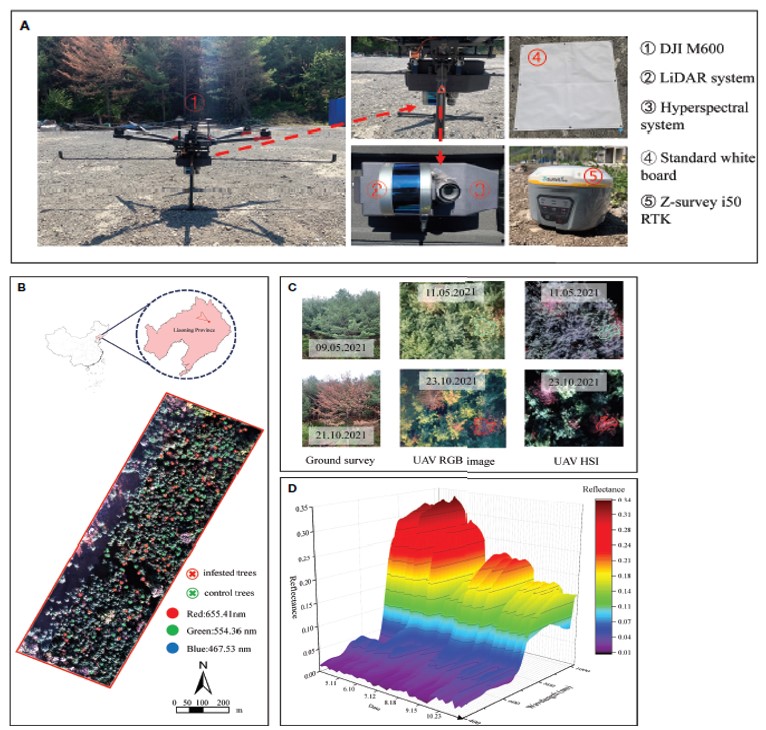
The UAV-based hyperspectral and LiDAR system (A) and location of the study area and the hyperspectral image of the test site acquired on 23 October (B). An example pine tree shows the process of discoloration in the field, UAV RGB and hyperspectral images (C) and examples of hyperspectral curves of sample trees at different dates (D).
【Results】
The results showed that: (1) the responses of the tree crown spectral features to PWD infestation could be detected before symptoms were noticeable in RGB data and field surveys; (2) the spectral derivatives were the most discriminable variables, followed by spectral reflectance and VIs; (3) based on the HSI data from July to October, the two groups of trees were successfully separated using the RF classifier, with an overall classification accuracy of 0.75-0.95.
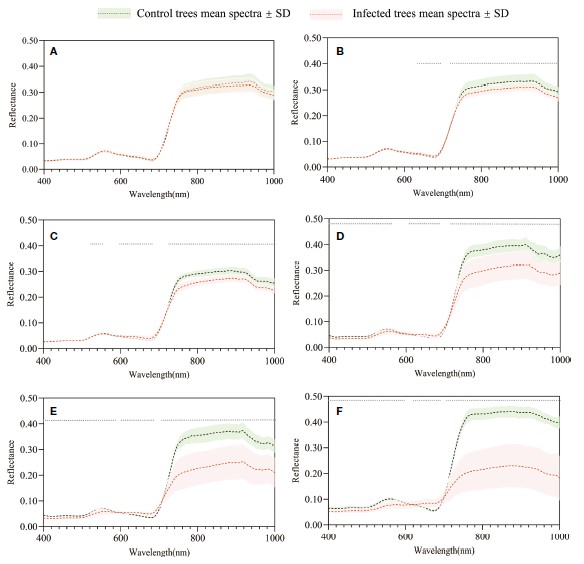
Mean spectra of infested and control trees of May (A), June (B), July (C), August (D), September (E), and October (F).
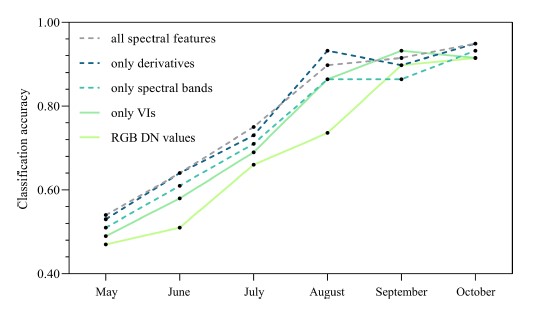
Classification accuracy for distinguishing infested and control trees by using different types of features during the course of pine wilt disease infestation.
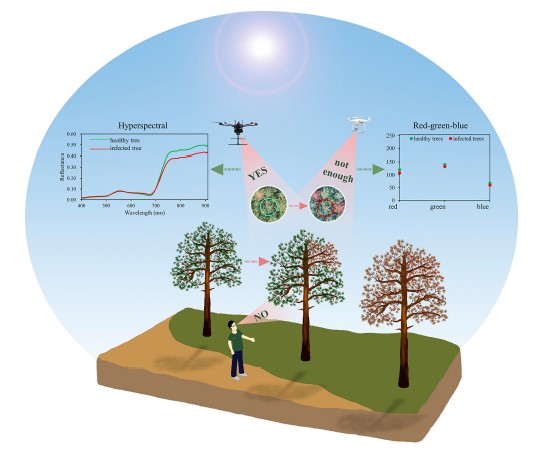
A schematic diagram of tree crown changes and the limited range of human observations.
【Conclusions】
The UAV-based RS data used in this study successfully detected changes in the spectral behavior of PWD-infested trees. Sample trees were monitored from May to October. In June, before changes were noticeable in the RGB data and field investigation, changes in spectral features were first detected in the hyperspectral data.
Several spectral features were employed to detect variations in the spectrum reaction of infested trees compared to non-infested trees. The spectral reflectance of infested trees was altered in the visible, red edge and NIR bands. The most discriminative features for separating the two groups’ trees were the derivatives and the spectral reflectance, over the REPs or VIs. By using the RF algorithm, the two groups were successfully separated from July to October.