Retrieving soil heavy metals concentrations based on GaoFen-5 hyperspectral satellite image
at an opencast coal mine, Inner Mongolia, China
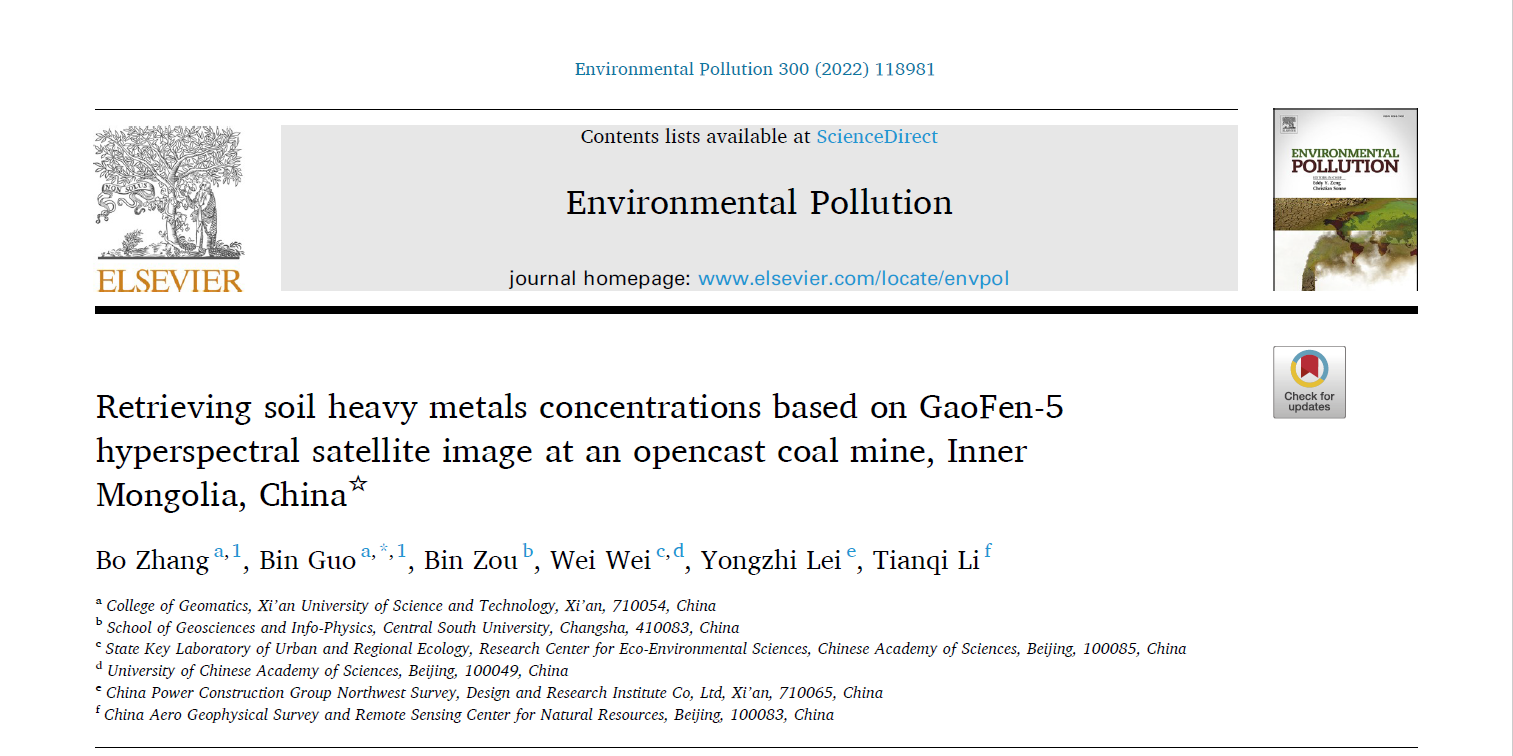
Soil heavy metals pollution has been becoming one of the severely environmental issues globally. Although mine resources development is vital for socioeconomic, some adverse effects generated by mine development on the natural environment were unavoidable. So, investigating soil heavy metals distribution in the open-pit coal mine is strongly desired because the results are meaningful for detecting contaminated fields, supplying land reclamation strategy, and further tactics of public health.
In-situ soil sampling combined with laboratory chemical analysis methods were widely used to survey and map the soil heavy metals concentrations. However, the continuous map of soil heavy metals concentrations was hardly obtained by the discrete sampling method and they were time consuming, expensive, and low efficiency. The remote sensing technology supplies a new perspective for convenient and economical monitoring heavy metals contents at large scales. However, some of the hyperspectral sensors used have problems such as poor data quality, limited image continuity, narrow spectral range, low spatial resolution, needing auxiliary environmental variables, and being susceptible to atmospheric interference. Fortunately, the GF-5 satellite launched on May 9, 2018, the first integrated hyperspectral satellite carrying Advanced Hyperspectral Imager (AHSI) for both land observations and atmospherics all over the world, supplied up-to-date datasets for earth science. The spatial resolution, the spectral resolution, the spectral range, and the time resolution of GF-5 AHSI were dramatically enhanced comparing with existed hyperspectral satellite sensors. However, fewer related studies in terms of retrieving soil heavy metals contents using GF-5 AHSI hyperspectral images have been reported.
Based on this, to fill these knowledge gaps, in this article, a research team from Xi’an University of Science and Technology retrieved soil heavy metals concentrations based on GaoFen-5 hyperspectral satellite image at an opencast coal mine. Aiming to (1) utilize a DS algorithm for establishing a transfer model of soil spectra between laboratory-measured and GF-5 AHSI obtained to improve the accuracy of the soil heavy metals concentrations estimation, and (2) map the soil heavy metals concentrations based on the optimal estimation model determined by comparing the random forest (RF), the extreme learning machine (ELM), the support vector machine (SVM), and the back-propagation neural network (BPNN) algorithms for the study area.
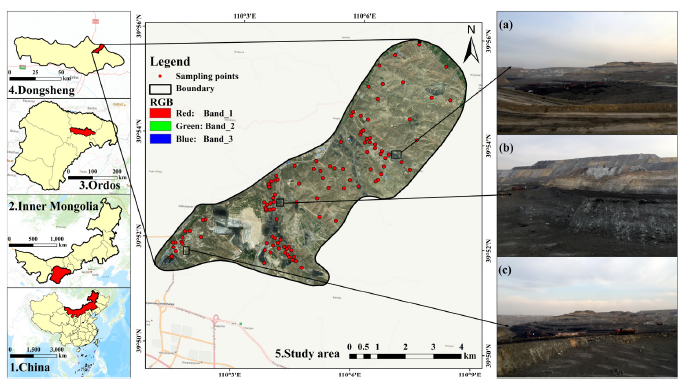
Fig. 1. The geographical location of the study area and the distribution of the in-situ soil samples. (a), (b), and (c) represent the field conditions of the study area.
The authors collected soil samples at 20 cm depth in February 2020, and measured the heavy metals concentrations and spectral data (ASD FieldSpec 4 Spectrometer) under laboratory conditions. The GF-5 AHSI imagery was obtained at 13:33:30 on 19 March of 2020. The direct standardization (DS) algorithm was introduced to calibrate the Gaofen-5 (GF-5) hyperspectral image based on the measured spectra of samples. The spectral reflectance of the GF-5 hyperspectral image was reconstructed using continuous wavelet transform (CWT) at different scales. The characteristic bands of GF-5 were selected by the Boruta algorithm. The RF, ELM, SVM, and BPNN algorithms were used to predict the heavy metals concentrations. The optimal model was determined by the coefficient of determination (R2), root means square error (RMSE), and means absolute error (MAE). Finally, the spatial distributions of soil heavy metals concentrations in this study were mapped.
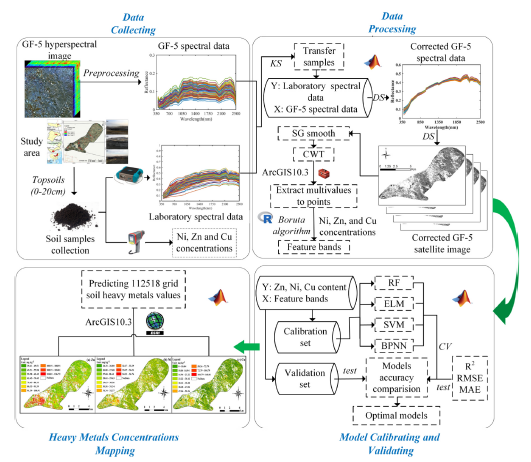
Fig. 2. Flowchart of the study.
[Results]
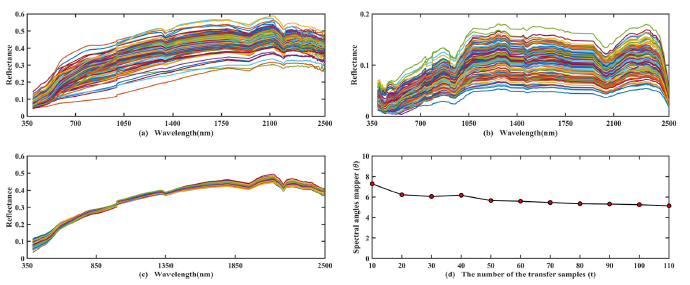
Fig. 3. Spectral reflectance curves of 110 soil samples and the evaluation of the DS algorithm in calibrating field spectra retrieved from GF-5 AHSI image. (a) laboratory spectra measured by ASD, (b) field spectra retrieved from GF-5 AHSI image, (c) field spectra calibrated by DS algorithm using laboratory spectra, and (d) spectral angles mapper (θ) used to evaluate the performance of the DS algorithm.
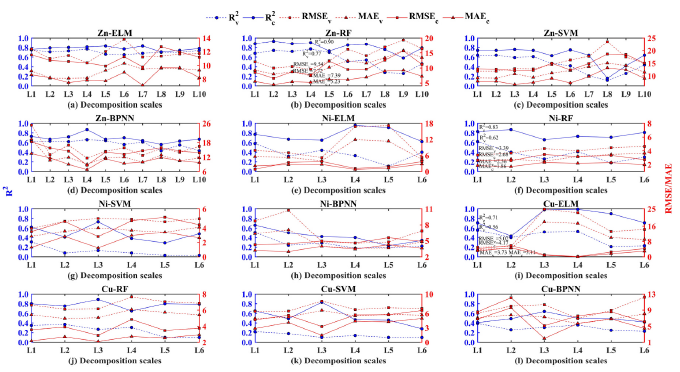
Fig.4. The accuracy evaluation of estimation models at each decomposition scale of CWT.
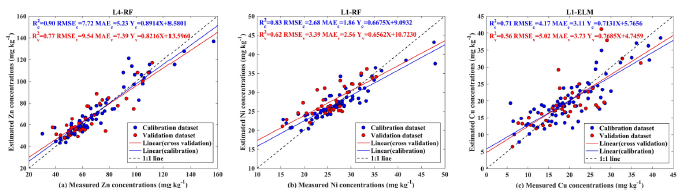
Fig.5. Scatter plots of the optimal estimation model for Zn, Ni, and Cu concentrations
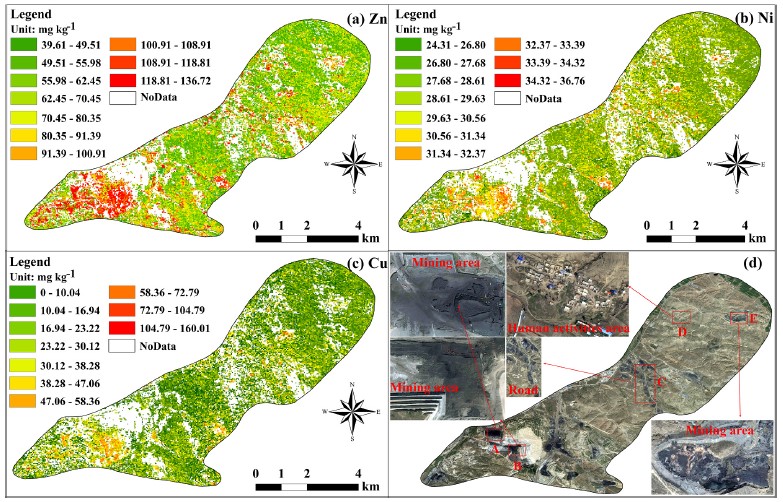
Fig. 6. The spatial distribution maps of heavy metals in the study area.
[Conclusions]
(1) CWT can effectively eliminate the noise of satellite hyperspectral data, and the Boruta algorithm can be used to extract the characteristic bands effectively. The Boruta algorithm eliminates redundant hyperspectral data and effectively retains the integrity of the hyperspectral data and the physical meaning of the original bands. The DS algorithm was feasible and reliable in calibrating GF-5 AHSI images for estimating heavy metals concentrations. The estimation accuracy was significantly improved by using the DS algorithm. For Zn, Ni, and Cu, the Rv2 were 0.77 (RF), 0.62 (RF), and 0.56 (ELM), respectively.
(2) The distribution trends of heavy metals were almost consistent with the results of actual ground measurements.
(3) The opencast coal mine activities may be the main source of soil heavy metals contamination in the mining area, and vehicle emissions, dust, wastewater, and garbage disposal may be other possible reasons for heavy metals pollution of the research area.
This paper revealed that the GF-5 can be one of the reliable satellite hyperspectral imagery for mapping soil heavy metals. The workflow and methods proposed by the current study can supply scientific support for estimating soil heavy metals concentrations at continuous scales.