Hyperspectral remote sensing to assess weed competitiveness in maize farmland ecosystems
Weeds are plants that crowd out maize crops, which affect the normal growth of maize by competing for nutrients, sunlight, space, and water. However, maintaining appropriate weed diversity has ecological functions such as controlling agricultural pests, maintaining soil, and promoting nutrient cycling. To better control weed damage, the competitive roles between weeds and crops are identified for differentiated ecological weed management. After the three-leaf stage, maize relies on the absorption of nutrients and water by the roots and the synthesis of organic matter by photosynthesis in the leaves. During this period, crop weeds began to compete for resources, but competition was not obvious, because sufficient resources were present. The intensity of competition gradually increases with the continuous growth of crops and weeds, and therefore the study of maize-weed competition is focused on the late maize seedling stage. Weed competition leads to changes in the morphological structure and physiology of the crop, including plant height, leaf area, nutrient uptake, and reduced early photosynthetic rates. Weed competition causes serious economic losses to maize production. Timely and accurate assessment of pressure from competition is crucial for ecological weed management. hyperspectral remote sensing or imagery can rapidly, reliably, and non-destructively evaluate the response of plants to stress factors, such as competition, diseases and insect pests, water shortage and salt stress. The theoretical basis is that weed competition causes changes in plant physiology that alter light absorption and canopy reflection properties. The combination of UAV remote sensing and deep learning provides an opportunity to quantify competitive responses at large scales.
Based on this, in the attached article, a group of scientists from Northeast Agricultural University applied hyperspectral remote sensing (HRS) technology to conduct a competitive experiment at the Xiang yang demonstration site of Northeast Agricultural University in Harbin, China (45°45′N, 126°54′E) from May to September 2021. The specific objectives are (i) revealing the mechanistic relationship between weed competition and maize structural and physiological information, and designing a CCI to express and quantify maize-weed competition characteristics; (ii) using HRS to reveal the competitive relationships of fine farmland communities and locate their sensitive spectral regions; and (iii) implementing early weed competition prediction in maize using a 3D-CNN deep learning model.
Maize was sown at a constant density in the experimental area on May 6, 2021. The experimental area is divided into a model training area and validation area. Weed density (set by random density survey in the experimental area) was set to 5 treatments (Levels 1–5, N0-N4): 0 plants/cm2, 20 plants/cm2, 40 plants/cm2, 80 plants/cm2, and 160 plants/cm2. Each treatment was replicated three times, with a total of 15 plots. Spectral data were collected under sunny weather conditions on June 12, 2021 using DJ M600 Pro UAV with LR1601-IRIS LIDAR (LR1601-IRIS, IRIS Inc., Beijing LICA United Technology Limited) and Pika L hyperspectral system (Resonon, USA). The ground truth data, included maize phenotypic data (plant height, stalk thickness) and physiological information (stomata, fluorescence, nutrients), as well as information on the coordinates of sampling site locations, are measured simultaneously. Then, designed CCI to express and quantify maize-weed competition characteristics. Finally, conducted spectral preprocessing, dataset construction, 3D-CNN network model development, model implementation and application.
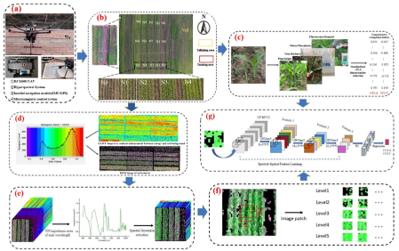
Spectral image processing, including (a) spectral collection equipment, (b) experimental area design, (c) comprehensive competition index design, (d) image preprocessing to remove the soil background, (e) hyperspectral dimensionality reduction, (f) dataset cutting, and (g) deep learning network model.
Results:
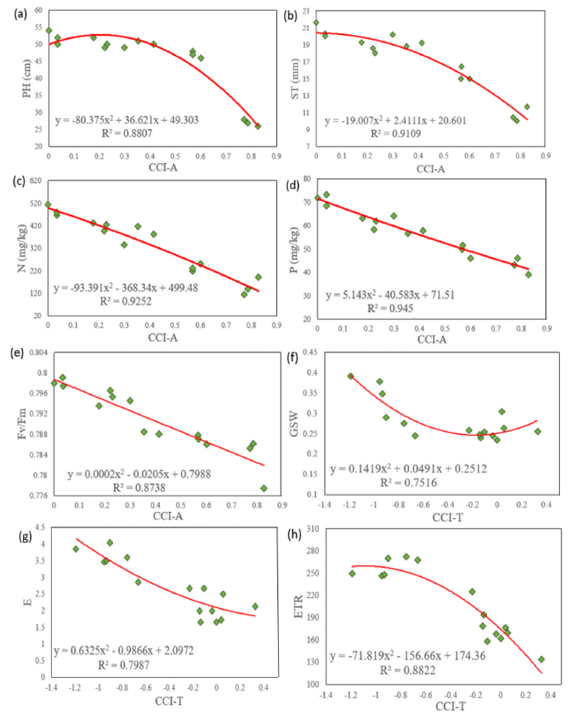
Regression relationship between CCI-A, CCI-T and maize parameters in structure, physiological and biochemical. (a) Plant height; (b) Stem thickness; (c) Nitrate; (d) Phosphorus; (e) Photosynthetic rate; (f) Stomatal conductance; (g) Transpiration rate; (h) Electron transfer rate.
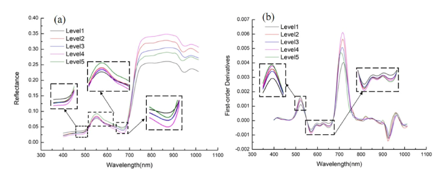
Spectral curve analysis. (a) Spectral reflectance curve of different competition levels; (b) Spectral first-order derivative curve of different competition levels.
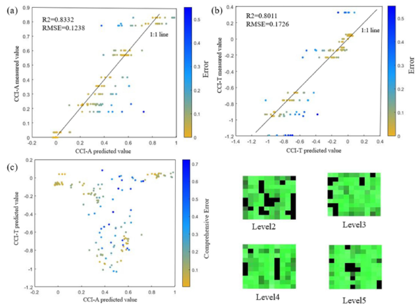
The prediction effect of the test set. (a) CCI-A prediction effect; (b) CCI-T prediction effect; (c) Comprehensive predicted effect of CCI-A and CCI-T.
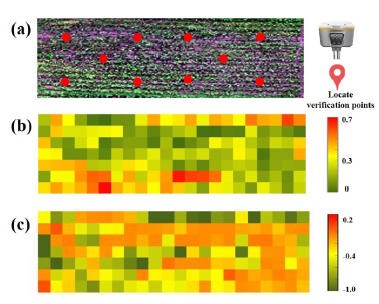
Model application effect. (a) Spectral image of the verification area; (b) Distribution map of CCI-A; (c) Distribution map of CCI-T.
Conclusions:
The designed competition indicators CCI-A and CCI-T can express and quantify the competitive characteristics of maize-weed from various aspects, such as morphological structure, physiology, and biochemistry. In particular, CCI-A has better adaptability to the expression of accumulative parameters than single RCI. However, CCI-T is influenced by many uncontrollable factors in the farm environment and has some fluctuations in the expression of physiological parameters.
The combination of the HRS and 3D-CNN model achieves high-throughput nondestructive prediction of weed competition indicators. Satisfactory prediction accuracy at 5 competing levels (Levels 1–5) when using the 13 bands with the highest VIP scores indicates the stability of the proposed model. The combination of HRS and deep learning has great potential to predict weed stress in the field early. It can provide guidance for the timely and accurate restoration of crops before they are damaged by weeds, and avoid the impact of weed infestation on agroecosystems and crop growth.