Heavy metals in soil are harmful, and their migration and accumulation can seriously threaten ecological environmental security and human health. Arsenic (As) exhibits high neurotoxicity and teratogenicity. Much As is released into soil during human activities, including mining operations and industrial production activities. Determining the As concentration in soil quickly and accurately is important for As pollution assessment. The traditional heavy metal survey method aims to perform chemical property testing of soil samples collected in the field, which is a costly and time-consuming method due to the complex field sampling, sample processing and chemical analysis procedures. Hyperspectral remote sensing with a high spectral resolution, wide band range and continuous spectral information is a quickly developing technology that has been widely used in the estimation of soil heavy metal concentrations. However, existing soil heavy metal concentration estimation models based on hyperspectral data ignore the spatial non stationarity of the relationship between the soil spectrum and heavy metal concentration.
Based on this, a group of Chinese scientists from Capital Normal University chose the northeast district of Beijing, China (40°10′0″-40°15′30″ N, 116°58′4″-117°5′4″ E) as a case study area and proposed a novel model (geographically weighted extreme gradient boosting or GW-XGBoost model) combining geographically weighted regression (GWR) method with XGBoost algorithm for estimating soil heavy metal concentration based on the laboratory measured hyperspectral data (ASD FieldSpec 4 spectrometer with a wavelength range of 350 to 2500 nm and a spectrum resampling interval of 1 nm). And then assessed the effectiveness of the proposed model.
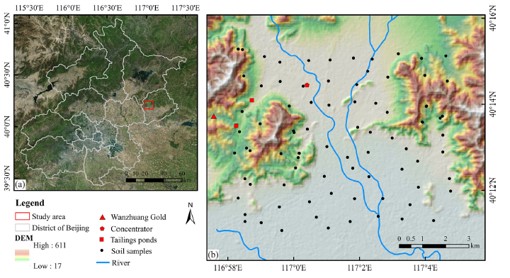
Location of study area and samples. (a) location of the study area in Beijing, China. The satellite image is from Bing; and (b) distribution of the samples, mine, tailing ponds and concentrator in the study area, which also shows the river and digital elevation model (DEM).
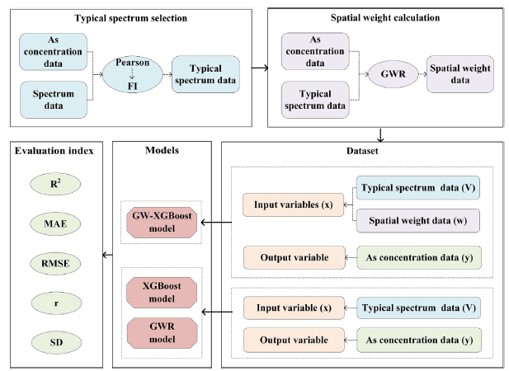
Flowchart of the As concentration estimation process.
Results:
Correlation diagram between As and spectrum. The shaded blocks indicate the main chemical absorption ranges.
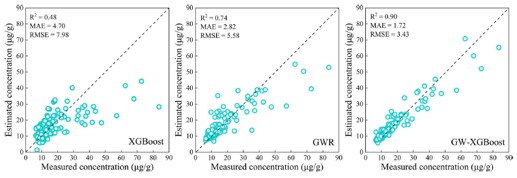
Scatter plots of the measured As concentration and estimated values via the proposed GW-XGBoost model and two validation models.
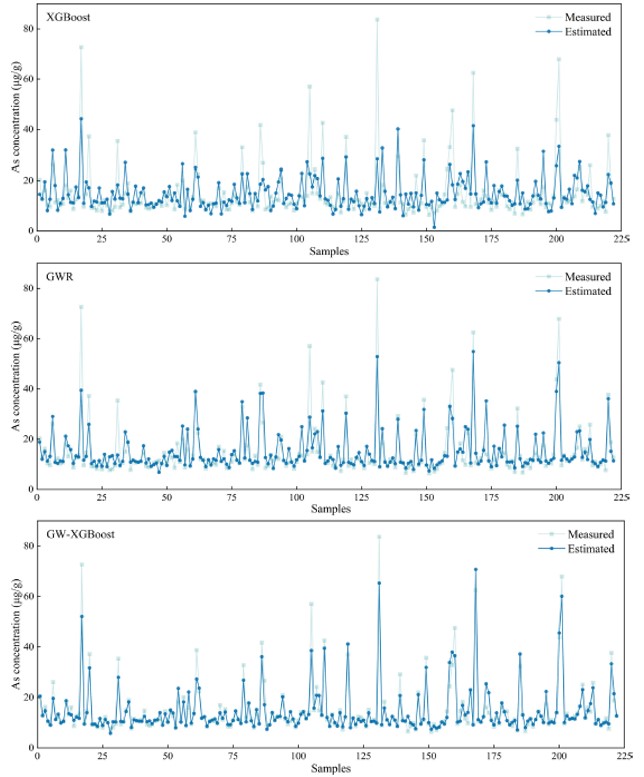
Comparison diagrams of the fit between the measured As concentration and estimated values via the proposed GW-XGBoost model and two validation models.
Conclusions:
The spectral bands chosen for the above As concentration estimation models were related to the absorption effects of spectrally active substances whose surface contains functional groups capable of forming complexes with As. Consideration of this adsorption mechanism in hyperspectral estimation model construction for heavy metal concentrations could effectively reduce the redundancy in hyperspectral data. The GW-XGBoost model effectively improved the estimation accuracy of the soil As concentration because it not only considers the heterogeneity of the relationship between As concentration and spectra but also considers their complex correlations. The GW-XGBoost model facilitated a more accurate estimation of soil heavy metal concentrations, which could provide technical support for large-scale monitoring of soil heavy metal pollution using hyperspectral technology.